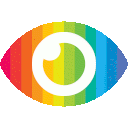
1. The study compares four data-mining techniques in predicting audit opinions on financial statements of companies listed on NYSE, AMEX, and NASDAQ from 2001 to 2017.
2. Decision Trees, Support Vector Machines, K-Nearest Neighbors, and Rough Sets were used to develop prediction models.
3. SVM models developed by the RBF kernel demonstrated the highest performance in terms of overall prediction accuracy rates and Type I and Type II errors.
The article titled "Audit Opinion Prediction: A Comparison of Data Mining Techniques" compares the effectiveness of four data-mining techniques in predicting audit opinions on companies' financial statements. The study uses a large dataset consisting of various financial and non-financial variables for U.S. companies listed on NYSE, AMEX, and NASDAQ from 2001 to 2017.
The article provides a detailed analysis of the four data-mining techniques used in the study, namely Decision Trees (DT), Support Vector Machines (SVM), K-Nearest Neighbors (K-NN), and Rough Sets (RS). The results indicate that all models developed using different algorithms demonstrate their highest performance in predicting going-concern modifications, ranging from 84.2 to 100 percent accuracy rates.
However, the article has some potential biases and limitations that need to be considered. Firstly, the study only focuses on U.S. companies listed on three stock exchanges, which may not be representative of all companies worldwide. Secondly, the article does not provide any information about the selection criteria for the variables used in the dataset or how they were collected. This lack of transparency raises questions about the reliability and validity of the results.
Moreover, while the article claims that all models predict audit opinions with relatively high accuracy rates, it does not provide any evidence to support this claim or compare these rates with other studies' findings. Additionally, there is no discussion about possible risks associated with relying solely on data-mining techniques for audit opinion prediction or how these techniques can complement traditional auditing methods.
Furthermore, the article does not explore counterarguments or alternative perspectives regarding data-mining techniques' effectiveness in predicting audit opinions. It also lacks a critical analysis of potential ethical concerns related to using such techniques in auditing practices.
In conclusion, while this article provides valuable insights into comparing different data-mining techniques' effectiveness in predicting audit opinions, it has some limitations and biases that need to be considered. Future research should address these limitations and provide a more comprehensive analysis of the potential risks and benefits of using data-mining techniques in auditing practices.