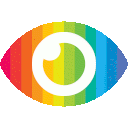
1. Exponential smoothing methods are widely used for univariate time series analysis due to their simplicity and robustness.
2. Multivariate exponential smoothing models can improve predictions of dependent time series by borrowing information from other series.
3. The Bayesian analysis of a general multivariate linear innovations state space model can be approached using MCMC simulation techniques, allowing for improved forecasting accuracy and reduced computing time.
The article "Forecasting correlated time series with exponential smoothing models" provides an overview of the use of exponential smoothing methods for forecasting univariate and multivariate time series. The article highlights the advantages of using multivariate time series models for dependent time series, where information can be borrowed from one series to improve predictions of another. The article also discusses the Bayesian analysis of a general multivariate linear innovations state space model, which cannot be formulated as a traditional multivariate regression model.
Overall, the article provides a comprehensive overview of exponential smoothing methods and their applications in forecasting correlated time series. However, there are some potential biases and limitations to consider.
Firstly, the article focuses primarily on the advantages of using multivariate time series models without exploring potential drawbacks or limitations. While it is true that these models can improve forecast accuracy, they may also be more complex and computationally intensive than univariate models. Additionally, assumptions about the correlation structure between variables may not always hold in practice.
Secondly, while the article briefly mentions extensions to exponential smoothing models that allow for covariates or non-regular seasonal cycles, it does not explore these topics in depth. This could limit the applicability of these methods in certain contexts where such extensions may be necessary.
Finally, while the article presents a Bayesian approach to analyzing multivariate linear innovations state space models, it does not compare this approach to other methods or discuss potential limitations or challenges associated with Bayesian analysis.
In conclusion, while "Forecasting correlated time series with exponential smoothing models" provides a useful overview of exponential smoothing methods and their applications in forecasting correlated time series, readers should be aware of potential biases and limitations associated with these methods. Further research is needed to fully understand the strengths and weaknesses of these approaches in different contexts.