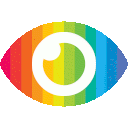
1. The fusion of medical imaging and electronic health records (EHR) using deep learning techniques can improve the accuracy and clinical relevance of diagnostic decisions.
2. Fusion strategies, such as early fusion, joint fusion, and late fusion, can be used to combine multiple modalities of data for better performance in medical imaging tasks.
3. Multimodal deep learning fusion models have shown improvements in accuracy and area under the receiver operating characteristic curve (AUROC) compared to single modality models in various medical applications.
The article titled "Fusion of medical imaging and electronic health records using deep learning: a systematic review and implementation guidelines" provides an overview of the use of deep learning techniques to combine medical imaging data with electronic health records (EHR) for improved diagnostic decision-making. While the article presents valuable information on the different fusion strategies and their applications in medical imaging, there are several potential biases and limitations that need to be considered.
One potential bias in the article is the focus on positive outcomes and improvements achieved through fusion techniques. The authors highlight that multimodal fusion models generally lead to increased accuracy and area under receiver operating characteristic curve (AUROC) compared to single modality models. However, it is important to note that not all studies included in the review showed significant improvements, and some even reported similar performance between fusion models and single modality models. This suggests that fusion techniques may not always be superior or necessary for all medical imaging tasks.
Another limitation of the article is its narrow focus on deep learning techniques for fusion, specifically convolutional neural networks (CNNs). While CNNs have shown promise in image recognition tasks, they may not be suitable or optimal for all types of medical imaging data. Other machine learning algorithms or hybrid approaches combining different types of models could also be explored but are not discussed in detail.
The article also lacks a comprehensive discussion on potential risks and limitations associated with fusion techniques. For example, there may be challenges related to data integration, interoperability, privacy concerns, and ethical considerations when combining sensitive patient information from EHR with medical imaging data. These issues should be addressed when implementing fusion techniques in clinical practice but are not adequately explored in the article.
Furthermore, the article does not provide a balanced view by discussing potential drawbacks or limitations of fusion techniques. It primarily focuses on the benefits and improvements achieved through fusion but fails to acknowledge any potential trade-offs or disadvantages. This one-sided reporting could lead readers to believe that fusion techniques are universally beneficial and should be adopted without considering the specific context or limitations.
Additionally, the article lacks a critical analysis of the quality and reliability of the studies included in the systematic review. While the authors mention that 17 studies were included, they do not provide information on the study design, sample size, methodology, or potential biases in these studies. Without this information, it is difficult to assess the strength of evidence supporting the claims made in the article.
In conclusion, while the article provides a comprehensive overview of fusion techniques for combining medical imaging and EHR data using deep learning, it has several limitations and biases that need to be considered. The focus on positive outcomes and improvements achieved through fusion techniques, lack of discussion on potential risks and limitations, one-sided reporting, and lack of critical analysis of included studies all contribute to a biased presentation of the topic. Further research and exploration are needed to fully understand the benefits and limitations of fusion techniques in medical imaging.