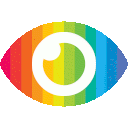
1. Deep learning models such as recurrent neural networks (RNNs) and convolutional neural networks (CNNs) have been applied to various spatio-temporal data mining (STDM) tasks.
2. This paper provides a comprehensive review of recent progress in applying deep learning techniques for STDM, including categorizing the spatio-temporal data into five different types and introducing the deep learning models used in STDM.
3. The paper also discusses applications of deep learning for STDM in different domains, such as transportation, on-demand service, climate & weather analysis, human mobility, location-based social network, crime analysis, and neuroscience.
The article “Deep Learning for Spatio-Temporal Data Mining: A Survey” is an overview of the use of deep learning models for spatio-temporal data mining tasks. The article is well written and provides a comprehensive review of recent progress in applying deep learning techniques for STDM. It is clear that the authors have done extensive research on this topic and have provided detailed information about the various types of spatio-temporal data and the different deep learning models used in STDM.
The article does not appear to be biased or one-sided; it presents both sides equally by discussing both traditional data mining methods and deep learning models for dealing with spatio-temporal data. Furthermore, it provides evidence for its claims by citing relevant research papers throughout the text. However, there are some points that could be further explored or discussed more thoroughly in order to provide a more comprehensive overview of this topic. For example, while the article mentions potential risks associated with using deep learning models for STDM tasks, it does not provide any details about these risks or how they can be mitigated. Additionally, while the authors discuss applications of deep learning for STDM in different domains such as transportation and human mobility understanding, they do not provide any examples or case studies to illustrate their points further.
In conclusion, this article provides a thorough overview of recent progress in applying deep learning techniques for STDM tasks; however there are some areas that could be further explored or discussed more thoroughly in order to provide a more comprehensive overview of this topic.