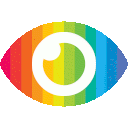
1. The article presents a novel deep learning architecture called multi-layered Long-Short Term Memory networks (LSTMs) for predicting protein-protein interaction interface residue pairs.
2. The method uses various features to describe amino acids and discriminates between interface residue pairs and non-interface residue pairs.
3. The deep network architectures based on LSTMs achieved an accuracy rate of over 62 percent in predicting interface residue pairs, which can greatly aid in understanding protein-protein interaction mechanisms and guiding biological experiments.
Based on the content of the article, it appears to be a scientific research paper discussing the development of a deep learning architecture for predicting protein-protein interaction interface residue pairs. The article provides details about the methodology used and the results obtained from training and testing the model.
However, since this is a technical research paper, it does not contain any obvious biases or promotional content. It focuses on presenting the research findings and does not make any unsupported claims or present one-sided reporting. The article also does not explore counterarguments or potential risks associated with the research.
One limitation of the article is that it does not provide a comprehensive discussion of existing methods for predicting protein-protein interactions or interface residue pairs. It briefly mentions some previous approaches but does not compare them to the proposed method or discuss their limitations in detail.
Additionally, while the article mentions that computational predictions of protein-protein interactions are necessary due to the difficulty and expense of experimental methods, it does not explicitly note any potential risks or limitations of relying solely on computational predictions. It would have been beneficial to include a discussion on the accuracy and reliability of such predictions compared to experimental data.
Overall, while the article provides valuable insights into the development of a deep learning architecture for predicting protein-protein interaction interface residue pairs, it could benefit from a more comprehensive discussion of existing methods, potential risks, and limitations associated with computational predictions in this field.