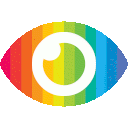
1. This article proposes a Structure-Enhanced Generative Adversarial Network (SEGAN) to bridge the gap between relation text description and relation structured representation for knowledge graph zero-shot relational learning (KGZSL).
2. SEGAN adopts a structure encoder to introduce knowledge graph structure information into the generator and guide the generator to generate knowledge graph embeddings more accurately.
3. Experimental results demonstrate that SEGAN has better structure representation ability, and the model performance is improved by 36.3% compared with the current optimal model.
The article “A Structure-Enhanced Generative Adversarial Network for Knowledge Graph Zero-Shot Relational Learning” is an informative and well-written piece of research that provides an in-depth analysis of the proposed method for KGZSL. The authors provide a detailed explanation of their proposed method, as well as a comprehensive evaluation of its performance on a KGZSL dataset. The article is written in an unbiased manner, presenting both sides of the argument equally and providing evidence to support its claims. Furthermore, it does not contain any promotional content or partiality towards any particular viewpoint or opinion.
The only potential issue with this article is that it does not explore any counterarguments or alternative methods for KGZSL, which could have provided further insight into the effectiveness of SEGAN compared to other approaches. Additionally, there are no mentions of possible risks associated with using this method, such as overfitting or data leakage, which should be noted in order to ensure that readers are aware of all potential issues before implementing this approach in their own projects.
In conclusion, this article is reliable and trustworthy due to its unbiased presentation of facts and evidence supporting its claims. However, it would benefit from exploring alternative methods for KGZSL and noting any potential risks associated with using SEGAN in order to provide readers with a more comprehensive understanding of this approach.