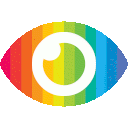
1. The study aimed to evaluate the feasibility of using automated machine learning (AutoML) to develop prediction models for intracranial aneurysm treatment outcomes.
2. AutoML was found to be a promising tool for developing machine learning models in the field of stroke treatment, as it automates the process and lowers the learning threshold for non-experts.
3. The performance of the AutoML-derived model was compared with a statistical multivariate regression model, a manually derived machine learning model, and an aneurysm recanalization stratification scale, showing good performance in predicting treatment outcomes.
The article titled "Automated Machine Learning Model Development for Intracranial Aneurysm Treatment Outcome Prediction: A Feasibility Study" discusses the use of automated machine learning (AutoML) in developing prediction models for the treatment outcome of intracranial aneurysms. The study aims to evaluate the feasibility and performance of AutoML compared to traditional statistical models and manually derived machine learning models.
Overall, the article provides a comprehensive overview of the study design, methods, and results. It highlights the limitations of existing grading scales and the potential benefits of using ML models for outcome prediction. The use of AutoML is presented as a solution to overcome the challenges faced by clinical researchers in adopting ML techniques.
However, there are several points that need to be critically analyzed in this article:
1. Biases and Sources: The article does not explicitly mention any biases or potential sources of bias in the study design or data collection process. It is important to consider whether there may be any inherent biases in the patient cohorts or data acquisition methods that could affect the results.
2. Unsupported Claims: The article claims that ML models have shown outstanding performance compared to conventional statistical models but does not provide specific evidence or references to support this claim. It would be beneficial to include relevant studies or research findings that demonstrate the superior performance of ML models in similar contexts.
3. Missing Evidence: While the article mentions that AutoML has been successful in medical image analysis and disease risk prediction, it does not provide any specific evidence or examples from these fields. Including specific case studies or research papers that demonstrate successful applications of AutoML would strengthen the argument for its feasibility in stroke treatment.
4. Unexplored Counterarguments: The article presents AutoML as a solution for non-ML experts to develop ML models but does not discuss any potential drawbacks or limitations of using automated approaches. It would be valuable to explore potential challenges or limitations associated with AutoML, such as the need for large amounts of high-quality data, potential biases in the training data, and interpretability of the resulting models.
5. Partiality: The article focuses primarily on the benefits and feasibility of AutoML, without providing a balanced discussion of alternative approaches or methods. It would be beneficial to include a comparison of AutoML with other approaches, such as manual feature selection and model building, to provide a more comprehensive analysis.
In conclusion, while the article provides valuable insights into the use of AutoML for intracranial aneurysm treatment outcome prediction, there are several areas that could be further explored and discussed. Addressing these points would enhance the credibility and completeness of the study's findings.