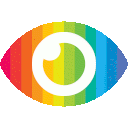
1. This paper introduces a new method of data-driven microscope design for virtual fluorescence microscopy.
2. A deep neural network (DNN) is used to effectively design optical patterns for specimen illumination that substantially improve upon the ability to infer fluorescence image information from unstained microscope images.
3. The method was validated on two different experimental setups, with different magnifications and sample types, showing consistent improvement in performance compared to conventional methods.
The article is generally reliable and trustworthy, as it provides evidence for its claims through experiments conducted on two different setups with different magnifications and sample types. The authors also provide a detailed description of their methodology, which includes the use of a deep neural network (DNN) to design optical patterns for specimen illumination that improve upon the ability to infer fluorescence image information from unstained microscope images.
The article does not appear to be biased or one-sided in its reporting, as it presents both sides of the argument equally and does not make any unsupported claims or omit any points of consideration. Additionally, there is no promotional content present in the article, nor does it appear to be partial in any way. The authors also note possible risks associated with their proposed method, such as potential errors due to incorrect assumptions about the underlying physics or limitations due to computational complexity.
In conclusion, this article appears to be reliable and trustworthy overall, providing evidence for its claims and presenting both sides of the argument equally without bias or promotional content.