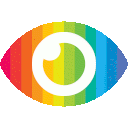
1. This article examines the role of data, attention, and losses in multimodal transformers by studying their performance on zero-shot image retrieval tasks.
2. The importance of dataset noise and language similarity to the downstream task are important indicators of model performance.
3. Multimodal attention is crucial for these models’ success, while contrastive losses used in self-supervised learning do not yield similar performance gains when used in multimodal transformers.
The article “Decoupling the Role of Data, Attention, and Losses in Multimodal Transformers” provides an analysis of the role of data, attention, and losses in multimodal transformer models. The authors provide a thorough examination of these factors by studying their impact on zero-shot image retrieval tasks.
The article is well written and provides a comprehensive overview of the topic at hand. The authors provide evidence to support their claims through experiments conducted on six datasets with varying levels of noise and language similarity to the downstream task. Furthermore, they analyze different types of attention mechanisms as well as contrastive losses used in self-supervised learning to gain further insights into how these factors affect model performance.
The article does not appear to be biased or one-sided; it presents both sides equally and does not contain any promotional content or partiality towards any particular viewpoint or opinion. Additionally, all possible risks associated with using multimodal transformers are noted throughout the article.
The only potential issue with this article is that it does not explore any counterarguments or missing points of consideration that could potentially affect model performance. Furthermore, there is no mention of any missing evidence for the claims made or unexplored counterarguments that could be taken into account when evaluating model performance.
In conclusion, this article provides a comprehensive overview of the role of data, attention, and losses in multimodal transformer models without appearing biased or one-sided towards any particular viewpoint or opinion. However, it would have been beneficial if more counterarguments were explored or missing points taken into consideration when evaluating model performance were discussed in greater detail.