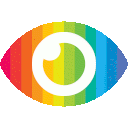
1. The study compares machine translation (MT) and human translation (HT) in terms of metadiscoursal interactional features using a parallel corpus of 79 texts translated from Chinese to English.
2. The statistical analysis shows no significant difference between Baidu and Google translate regarding all types of metadiscoursal indicators, but there are disparities in the interactional characteristics of various HT and MT groups.
3. Human translators outperform machine translations in the use of attitude markers, while the distribution of directives in machine-translated texts is more native-like. Both MT and HT use significantly fewer hedges, self-mention, and readers than non-translated texts.
The article "Automated and Human Interaction in Written Discourse: A Contrastive Parallel Corpus-based Investigation of Metadiscourse Features in Machine-Human Translations" presents a study that compares the metadiscoursal interactional features of machine translation (MT) and human translation (HT). The authors use a parallel corpus consisting of 79 texts translated from Chinese to English by professional human translators and machine translations (Baidu translate & Google translate) and a comparable reference corpus of non-translated English text.
The study finds no statistically significant difference between Baidu and Google translate regarding all types of metadiscoursal indicators. However, the authors note significant disparities in the interactional characteristics of various HT and MT groups. Compared to the metadiscourse features in non-translated English political texts, human translators were found to outperform machine translations in the use of attitude markers. In contrast, the distribution of directives in machine-translated texts is more native-like. Additionally, both MT and HT have utilized a significantly smaller number of hedges, self-mention, and readers than non-translated texts.
Overall, the article provides valuable insights into the differences between MT and HT regarding metadiscoursal interactional features. However, there are some potential biases and limitations to consider.
Firstly, the study only focuses on Chinese to English translations, which limits its generalizability to other language pairs. Additionally, it only considers political texts, which may not be representative of other genres or domains.
Secondly, while the study finds no statistically significant difference between Baidu and Google translate regarding all types of metadiscoursal indicators, it does not explore potential differences in accuracy or fluency between these two MT systems.
Thirdly, while the authors note significant disparities in the interactional characteristics of various HT and MT groups, they do not provide an explanation for why these differences exist or how they may impact translation quality.
Finally, while the article notes that both MT and HT have utilized a significantly smaller number of hedges, self-mention, and readers than non-translated texts, it does not explore why this may be the case or how it may impact reader perception or understanding.
In conclusion, while "Automated and Human Interaction in Written Discourse" provides valuable insights into differences between MT and HT regarding metadiscoursal interactional features for Chinese to English political texts specifically, there are potential biases and limitations to consider. Further research is needed to explore these issues more comprehensively across different languages and genres/domains.