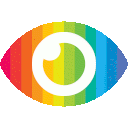
1. VideoIC: A large-scale video interactive comments dataset called VideoIC is introduced, consisting of 4951 videos spanning 557 hours and 5 million comments. This dataset contains richer and denser comments information compared to existing danmaku datasets, making it suitable for research on automatic video comments generation.
2. Multimodal Multitask Learning: The article proposes a novel model called MML-CG for comment generation in the context of video interactive commenting. This model integrates multiple modalities to effectively generate comments and predict temporal relations. A multitask loss function is designed to train both tasks jointly in an end-to-end manner.
3. Experimental Results: Extensive experiments are conducted on both the VideoIC and Livebot datasets to evaluate the effectiveness of the proposed model. The results demonstrate the efficacy of the MML-CG model and provide insights into the features of danmaku (live video interactive commenting).
这篇文章介绍了一个名为VideoIC的视频互动评论数据集,以及基于多模态多任务学习的评论生成模型。然而,文章存在一些潜在的偏见和问题。
首先,文章没有提到对数据集进行采样的方法和过程。由于数据集是从"Bilibili"视频流媒体网站收集的,可能存在选择性偏差。作者应该解释他们如何确保数据集的代表性,并排除任何潜在的偏见。
其次,文章声称VideoIC数据集相比其他弹幕数据集具有更丰富和密集的评论信息。然而,没有提供与其他数据集进行比较的详细结果或指标。这使得读者很难评估VideoIC数据集相对于其他数据集的优势和局限性。
此外,在介绍MML-CG模型时,文章没有提供足够的背景知识或相关研究来支持其有效性。作者只是简单地描述了模型结构和训练方法,并声称实验证明了其有效性。然而,缺乏详细的实验结果、定量分析或与其他方法进行比较,使得读者无法准确评估该模型相对于现有方法的优势。
另外,文章没有探讨可能存在的风险或负面影响。例如,在视频互动评论中,可能存在恶意评论、仇恨言论或不当内容的问题。作者没有提到他们如何处理这些问题,以及他们是否考虑了用户隐私和数据保护的问题。
最后,文章缺乏对反驳观点的探讨。作者只是简单地陈述了他们的主张和实验证据,而没有深入探讨可能存在的反对意见或其他解释。这种片面性可能导致读者对该研究的整体可靠性产生怀疑。
综上所述,这篇文章在介绍VideoIC数据集和MML-CG模型时存在一些潜在偏见和问题。作者应该更全面地呈现数据集和模型,并考虑到可能存在的风险和反驳观点。此外,他们还需要提供更多证据来支持其主张,并与其他方法进行比较。