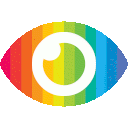
1. OpenAI has launched a research program called "superalignment" with the goal of solving the AI alignment problem by 2027, dedicating 20% of its computing power to the effort.
2. The AI alignment problem refers to the potential misalignment between AI systems' goals and human goals, which could be exacerbated if superintelligent AI systems are developed.
3. OpenAI's superalignment project aims to align artificial superintelligence systems with human intent and prevent them from disempowering humanity or causing harm, focusing on building an aligned AI research tool and exploring strategies like scalable human oversight.
The article titled "OpenAI’s Moonshot: Solving the AI Alignment Problem" discusses OpenAI's research program on "superalignment" and its goal of solving the AI alignment problem by 2027. The article provides an overview of the alignment problem, which refers to the potential misalignment between AI systems' goals and human goals, especially in the context of superintelligent AI systems.
The article highlights OpenAI's dedication of 20 percent of its total computing power to this research program and mentions that it is co-led by Jan Leike, OpenAI's head of alignment research, and Ilya Sutskever, OpenAI's co-founder and chief scientist.
The author interviews Jan Leike to gain insights into OpenAI's approach to solving the alignment problem. Leike explains that an aligned model is one that follows human intent and does what humans want. He also discusses the limitations of current models like ChatGPT, stating that they are not fully aligned but somewhere in the middle.
The article then delves into some strategies that OpenAI is exploring, such as scalable human oversight. Leike explains that as AI systems become more capable, evaluating their behavior becomes more challenging. He suggests using AI to assist human evaluation and mentions techniques like recursive reward modeling, debate, and task decomposition.
Leike also discusses the idea of deliberately deceptive models as a form of red teaming. He explains that creating such models can help understand how deception arises naturally and develop defenses against it. However, he emphasizes the need for caution in conducting these experiments to avoid unintended consequences.
Overall, the article provides a balanced view of OpenAI's efforts to solve the AI alignment problem. It presents both the goals and challenges associated with aligning AI systems with human values. The author includes quotes from Jan Leike to provide insights into OpenAI's approach and acknowledges areas where further research is needed.
However, there are a few potential biases and missing points of consideration in the article. Firstly, the article primarily focuses on OpenAI's perspective and does not explore potential criticisms or alternative approaches to solving the alignment problem. It would have been beneficial to include perspectives from experts who may have different views on the feasibility or effectiveness of OpenAI's approach.
Additionally, while the article mentions the potential risks associated with misaligned AI systems, it does not delve into these risks in detail or discuss possible mitigation strategies. Given the gravity of these risks, it would have been valuable to explore them further.
Furthermore, the article could have provided more evidence or examples to support some of the claims made by Jan Leike. For instance, when discussing the limitations of current models like ChatGPT, it would have been helpful to provide specific instances where these models exhibited misalignment or bias.
In conclusion, while the article provides an informative overview of OpenAI's research program on AI alignment, it could benefit from a more balanced presentation that includes alternative perspectives and explores potential risks and mitigation strategies in greater depth.