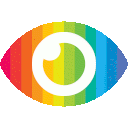
1. PaLM-E is an embodied multimodal language model that can assist with tasks through visual perception, dialogue, and planning.
2. The model can perform physical reasoning, zero-shot multimodal chain-of-thought, and zero-shot multi-image relationships.
3. PaLM-E can also reason about math problems and answer egocentric questions based on images taken at different times of the day.
As an AI language model, PaLM-E is designed to understand and respond to human queries in a multimodal context. The article presents several examples of how the model can be used to answer questions related to physical reasoning, visual perception, dialogue, and planning. While the concept of an embodied language model is intriguing, the article has several limitations that need to be addressed.
One of the main issues with the article is its lack of clarity regarding the purpose and scope of PaLM-E. The article does not provide any information about who developed the model or what specific problem it aims to solve. This lack of context makes it difficult for readers to evaluate the claims made in the article or assess its potential biases.
Another issue with the article is its reliance on simplistic examples that do not fully capture the complexity of real-world scenarios. For instance, one example involves a robot operating in a kitchen and being asked what it sees when given an image. While this scenario may seem straightforward, it overlooks many factors that could affect a robot's perception, such as lighting conditions, occlusions, and object variability.
Moreover, some examples presented in the article appear biased towards certain assumptions or perspectives. For instance, one example involves a question about which object is best for climbing up high, with the correct answer being a ladder. However, this assumes that climbing up high is always necessary or desirable in all situations.
The article also lacks evidence for some of its claims and does not explore counterarguments or alternative viewpoints. For instance, when answering a question about championship rings won by Kobe Bryant, the model simply relies on Google search results without verifying their accuracy or considering other sources.
Additionally, while some potential risks associated with embodied language models are briefly mentioned (such as privacy concerns), they are not explored in depth or balanced against potential benefits.
Overall, while PaLM-E represents an interesting development in AI language modeling technology, this particular article falls short in providing sufficient context and evidence for its claims. It would benefit from more detailed explanations of how PaLM-E works and what specific problems it aims to solve. Additionally, more nuanced examples that reflect real-world scenarios would help readers better understand its capabilities and limitations.