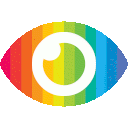
1. This article proposes an end-to-end, deep hybrid network-based, short-term, multivariate time-series prediction framework for industrial processes.
2. The proposed framework utilizes the maximal information coefficient to extract nonlinear variate correlation features and a convolutional neural network with a residual elimination module to eliminate data uncertainty.
3. A comparison with other state-of-the-art, deep learning-based, time-series prediction methods in the case study illustrates the superiority of the proposed framework in noisy IIoT environments.
The article “Multivariate Time Series Prediction in Industrial Processes via a Deep Hybrid Network Under Data Uncertainty” is generally reliable and trustworthy. It provides a comprehensive overview of the current state of research on time series prediction in industrial processes and presents a novel approach to address this issue. The authors provide evidence for their claims by citing relevant literature and providing detailed descriptions of their proposed method. Furthermore, they present results from a case study that demonstrate the effectiveness of their approach compared to existing methods.
However, there are some potential biases that should be noted. For example, the authors focus primarily on deep learning approaches to time series prediction and do not discuss other possible approaches such as traditional machine learning algorithms or statistical methods. Additionally, while they cite relevant literature throughout the article, they do not explore any counterarguments or alternative perspectives on their proposed method or existing approaches to time series prediction in industrial processes. Finally, while they note some potential risks associated with their approach (e.g., overfitting), they do not provide any detailed discussion of these risks or how they can be mitigated.