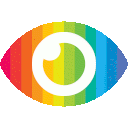
1. Robot-assisted minimally invasive surgery (RMIS) has advantages over traditional open surgeries, such as less pain and quicker recovery. However, the narrow field of view and intensive workload for surgeons in RMIS make automatic context awareness crucial for improving surgeon performance and patient safety.
2. Automatic segmentation of surgical instruments is challenging due to factors like motion blur, specular reflection, tissue occlusion, and limited endoscopic view. Previous methods using hand-crafted features and machine learning classifiers were ineffective in distinguishing specific parts and types of instruments.
3. Deep learning techniques, particularly convolutional neural networks (CNNs), have shown promise in accurate segmentation of surgical instruments. The proposed model in this study combines the Mask R-CNN framework with anchor optimization and an improved Region Proposal Network (RPN) to achieve accurate instance segmentation of surgical instruments. Cross-dataset evaluation demonstrates the model's generalization capability and its potential for improving performance on in-house datasets.
这篇文章主要介绍了在机器人手术中准确实例分割手术器械的方法和模型改进,并对跨数据集进行评估。然而,文章存在一些问题和偏见。
首先,文章没有提及任何可能的风险或局限性。机器人手术是一项复杂的技术,可能存在许多潜在的风险和挑战,如系统故障、误操作等。文章应该更加平衡地呈现这些问题,并提供解决方案或建议。
其次,文章没有充分考虑到实际临床环境中的因素。机器人手术通常在狭小的工作空间中进行,而且手术器械可能会受到组织遮挡、光照变化等因素的影响。作者应该更加关注这些实际问题,并提出相应的解决方案。
此外,文章没有提供足够的证据来支持其所提出的主张。虽然作者声称他们的模型在不同数据集上表现良好,但缺乏详细的实验证据来支持这一观点。读者很难判断该模型是否真正具有广泛适用性和鲁棒性。
最后,文章可能存在宣传内容和偏袒的问题。作者提到了一些先前研究的进展,但没有充分讨论其他可能的方法或模型。这可能导致读者对该领域的整体了解不足,并且无法全面评估该模型的优势和局限性。
综上所述,这篇文章在介绍机器人手术中手术器械实例分割的方法和模型改进方面提供了一些有用的信息,但存在一些偏见和不足之处。作者应该更加平衡地呈现问题,并提供更多实验证据来支持其主张。此外,他们还应该考虑到实际临床环境中的因素,并探索其他可能的方法和模型。