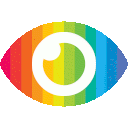
1. Stock price prediction mechanisms are fundamental to the formation of investment strategies and the development of risk management models.
2. Computational advances have led to several machine learning algorithms used to anticipate market movements consistently and thus estimate future asset values such as company stock prices.
3. Support Vector Regression (SVR) is among the most widely used techniques for predicting stock prices, and different frequencies of data can be used for predictions, including daily and up-to-the-minute prices.
The article titled "Stock price prediction using support vector regression on daily and up to the minute prices" provides an overview of the use of machine learning algorithms, specifically Support Vector Regression (SVR), in predicting stock prices. The article highlights the challenges faced when developing price forecasting systems due to the peculiarities of financial time series and the Efficient Market Hypothesis (EMH), which states that it is not possible to consistently obtain risk-adjusted returns above the profitability of the market as a whole.
The article presents a brief literature review on EMH and its limitations, highlighting studies that have shown that stock market prices are predictable to some extent. However, there is no discussion on studies that have found evidence supporting EMH or counterarguments against predictive systems.
The article also discusses the use of SVM-based models in predicting stock prices and compares results obtained from daily data with those obtained from up-to-the-minute data. The study tests stock predictability in Brazil, China, and the United States based on prediction error analysis. However, there is no discussion on potential biases in selecting these countries or how their markets differ from others.
The article concludes by stating that strategies based on price prediction may provide evidence against EMH and contribute to building profitable strategies. However, there is no discussion on potential risks associated with relying solely on predictive models for investment decisions or how these models may be affected by changes in market conditions.
Overall, while the article provides useful insights into using machine learning algorithms for predicting stock prices, it lacks a balanced perspective and does not address potential biases or limitations in its approach.