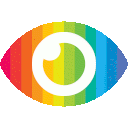
1. Digital footprints can be used to identify opinion leaders (OLs) in a more efficient and cost-effective way than traditional survey-based approaches.
2. The attributes "what one knows" and "how active one is" are the best predictors for identifying OLs based on their digital footprints on Facebook.
3. This approach allows for an automated identification of OLs, but also raises concerns about data privacy and ethical considerations.
The article "Inferring opinion leadership from digital footprints" discusses the use of digital footprints to identify opinion leaders in online social networks. The authors argue that traditional survey-based approaches to identifying opinion leaders are limited in sample size and time-consuming, and propose a more efficient and cost-effective approach using digital footprints.
The article provides a thorough review of the theoretical background of opinion leadership and its importance in various fields such as marketing, politics, and finance. It also discusses the potential ethical concerns surrounding the use of digital footprints for predicting personal attributes, citing the example of Cambridge Analytica's involvement in political campaigns.
The authors conducted a study using Facebook data to identify opinion leaders based on their digital footprints. They found that "what one knows" and "how active one is" were the best predictors for identifying opinion leaders. However, the article does not provide enough information about how these predictors were operationalized or how they were linked to the established opinion leadership scale used in the study.
One potential bias in this article is its focus on Facebook as the primary source of data for identifying opinion leaders. While Facebook remains popular, it may not be representative of all online social networks or user behaviors. Additionally, the study only included 272 users, which may not be sufficient to draw generalizable conclusions about identifying opinion leaders through digital footprints.
Another limitation of this article is its lack of discussion on potential risks associated with using digital footprints for predicting personal attributes. While the authors briefly mention ethical concerns related to Cambridge Analytica's activities, they do not explore other possible risks such as privacy violations or algorithmic biases.
Overall, while this article provides an interesting perspective on using digital footprints to identify opinion leaders, it would benefit from more detailed explanations of its methodology and limitations. Additionally, it should consider potential biases and risks associated with this approach more thoroughly.