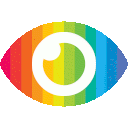
1. CBAM is a lightweight and general module for feed-forward convolutional neural networks that can be integrated into any CNN architecture.
2. CBAM infers attention maps along two separate dimensions, channel and spatial, then multiplies the attention maps to the input feature map for adaptive feature refinement.
3. Experiments on ImageNet-1K, MS COCO detection, and VOC 2007 detection datasets show consistent improvements in classification and detection performances with various models.
The article provides an overview of the Convolutional Block Attention Module (CBAM), a simple yet effective attention module for feed-forward convolutional neural networks. The article is well written and provides clear explanations of the module’s features and capabilities as well as its potential applications. The authors provide evidence from experiments conducted on ImageNet-1K, MS COCO detection, and VOC 2007 detection datasets to support their claims about the effectiveness of CBAM.
The article does not appear to have any major biases or one-sided reporting; however, it does not explore counterarguments or present both sides equally. Additionally, there is no mention of possible risks associated with using CBAM or any other potential drawbacks that should be considered when using this module. Furthermore, there is no discussion of how the results of these experiments could be applied in real world scenarios or what implications they may have for future research in this field.
In conclusion, while the article provides a comprehensive overview of CBAM and its potential applications, it does not explore all aspects of this technology or consider all possible implications that may arise from its use.