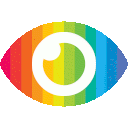
1. Financial cybercrime is a combination of financial crime, hacking, and social engineering committed over cyberspace for the sole purpose of illegal economic gain.
2. Machine learning and deep learning methods are being used to combat financial cybercrime, with group anomaly detection (GAD) being a key technique.
3. The article provides a comprehensive survey of the financial cybercrime ecosystem, including fraud methods adopted by criminals, relevant systems and algorithms, personas involved, and open and emerging problems in the domain.
The article "Financial Cybercrime: A Comprehensive Survey of Deep Learning Approaches to Tackle the Evolving Financial Crime Landscape" provides a comprehensive overview of the use of machine learning and deep learning methods in combating financial cybercrime. The authors define financial cybercrime as a combination of financial crime, hacking, and social engineering committed over cyberspace for illegal economic gain. They argue that traditional rule-based systems and shallow anomaly detection methods are insufficient to detect financial cybercrime, and that graph-based techniques and neural network models are needed.
The article is well-researched and provides a thorough analysis of the different fraud methods adopted by criminals, relevant systems, algorithms, drawbacks, constraints, and metrics used to combat each fraud type, relevant personas and stakeholders involved, and open and emerging problems in the financial cybercrime domain. The authors also provide a glossary for all abbreviations used throughout the survey.
However, there are some potential biases in the article. For example, the authors focus primarily on machine learning and deep learning approaches to combating financial cybercrime while neglecting other potential solutions such as increased regulation or improved cybersecurity measures. Additionally, while the authors acknowledge that there is a lack of transparency, fairness, and privacy from customers and regulators when it comes to applying artificial intelligence methods to detect fraud-related activities, they do not fully explore these concerns or potential risks associated with using these methods.
Overall, "Financial Cybercrime: A Comprehensive Survey of Deep Learning Approaches to Tackle the Evolving Financial Crime Landscape" is a valuable resource for those interested in understanding how machine learning and deep learning can be used to combat financial cybercrime. However, readers should be aware of potential biases in the article and consider other perspectives when evaluating solutions to this complex problem.