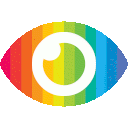
1. The current study used machine learning models based on single-trial electroencephalogram (EEG) to identify fragmented reading (FR) with high accuracy.
2. Alpha and lower gamma frequency bands were found to be important in identifying FR, with FR subjects showing increased alpha power in central and parieto-occipital sites and decreased gamma power in frontal sites.
3. This study provides quantitative and objective evidence of the negative effects of FR on cognitive abilities, such as attention and working memory, using neurophysiological techniques.
The article titled "Identifying Fragmented Reading and Evaluating Its Influence on Cognition Based on Single Trial Electroencephalogram" presents a study that aims to identify fragmented reading (FR) using machine learning models based on electroencephalogram (EEG) data. The study also investigates the influence of FR on cognition by analyzing EEG spectrum characteristics.
One potential bias in the article is the selection of subjects. The study only included college students from a single university in China, which may limit the generalizability of the findings. Additionally, the criteria for selecting subjects based on their time spent on social media apps may introduce bias as it assumes that more time spent on FR indicates a higher level of engagement or impairment.
The article claims that FR can lead to cognitive impairment, but it does not provide sufficient evidence to support this claim. While previous studies have suggested that FR can cause impatience, inattention, and difficulty in logical thinking, these studies are not directly related to cognitive impairment. The article would benefit from including more research specifically focused on cognitive impairment caused by FR.
Another limitation of the study is its reliance on ML models for identifying FR. While ML models can be useful for individual-level identification, they are not infallible and may introduce errors or biases in classification. It would be important to validate the accuracy of the ML models used in this study and consider potential limitations or sources of error.
The article also lacks exploration of counterarguments or alternative explanations for the observed effects of FR on cognition. It would be valuable to discuss other factors that could potentially influence cognitive abilities, such as sleep quality, stress levels, or overall mental health.
Furthermore, the article does not adequately address potential risks associated with FR or provide recommendations for mitigating these risks. Given that FR is becoming increasingly prevalent in society, it is important to consider both the positive and negative impacts it may have on individuals' well-being and cognitive functioning.
Overall, the article provides an interesting exploration of FR and its potential influence on cognition using EEG data. However, it would benefit from addressing the limitations and biases in subject selection, providing more evidence for the claims made, considering alternative explanations, and discussing potential risks and mitigation strategies associated with FR.