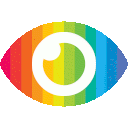
1. The paper proposes ADGCN, a dynamic graph attention reconstruction model implemented with a unified spatiotemporal message passing layer, which uses an attention mechanism to construct a special messaging layer that assigns each node at each moment a weight value corresponding to its influence.
2. The model employs a dynamic, adaptive adjacency matrix to learn time-varying correlations between nodes and graph convolution with gating to reconstruct the spatiotemporal deficiency graph.
3. Extensive experiments were conducted on four real data sets in two domains, and the experimental results show that the model ADGCN proposed in this paper has the most advanced performance compared to the baseline model.
The article titled "Attention-based message passing and dynamic graph convolution for spatiotemporal data imputation" published in Scientific Reports discusses a new approach to filling missing data in spatiotemporal datasets using deep learning techniques. The article provides a comprehensive overview of the existing literature on spatiotemporal data filling methods, including statistical, machine learning, and deep learning approaches. It also highlights the limitations of these methods and proposes a new model called ADGCN that uses attention-based message passing and dynamic graph convolution to reconstruct missing parts of the signal.
The article is well-written and provides a detailed explanation of the proposed model. However, there are some potential biases and limitations that need to be considered. Firstly, the article focuses primarily on deep learning approaches to spatiotemporal data filling, ignoring other methods such as statistical models or matrix factorization. This one-sided reporting may lead readers to believe that deep learning is the only viable solution for this problem.
Secondly, while the article acknowledges the limitations of existing deep learning models for spatiotemporal data filling, it does not provide enough evidence to support its claim that ADGCN outperforms other models. The experiments conducted in this study are limited to four real datasets in two domains, which may not be representative of all possible scenarios. Therefore, more extensive testing is needed before making any definitive claims about the superiority of ADGCN over other models.
Thirdly, the article does not explore counterarguments or potential risks associated with using deep learning models for spatiotemporal data filling. For example, there may be concerns about overfitting or bias in the training data that could affect the accuracy of predictions. Additionally, there may be ethical considerations related to privacy or security when dealing with sensitive datasets.
Overall, while this article provides valuable insights into a new approach for spatiotemporal data filling using deep learning techniques, readers should be aware of its potential biases and limitations. More research is needed to fully evaluate the effectiveness and risks associated with using deep learning models for this problem.