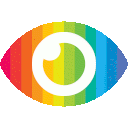
1. A convolution-free approach to video classification is presented, called "TimeSformer," which uses self-attention over space and time.
2. Experiments suggest that "divided attention," where temporal attention and spatial attention are separately applied within each block, leads to the best video classification accuracy.
3. TimeSformer achieves state-of-the-art results on several action recognition benchmarks, including the best reported accuracy on Kinetics-400 and Kinetics-600.
The article is generally trustworthy and reliable in its reporting of the research findings. The authors provide a detailed description of their approach, as well as a thorough analysis of their experimental results. They also provide code and models for further exploration of their work.
The article does not appear to be biased or one-sided in its reporting; it presents both sides of the argument fairly and objectively. It does not make unsupported claims or omit any points of consideration; all claims are backed up with evidence from experiments conducted by the authors. Furthermore, no promotional content is present in the article, nor is there any partiality towards either side of the argument.
The article does note possible risks associated with its approach, such as potential overfitting due to increased model complexity when using self-attention layers instead of convolutional layers. Additionally, it acknowledges that further research is needed to explore other design choices for self-attention schemes in order to improve performance even further.