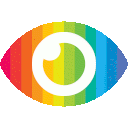
1. The article proposes a method for cancer gene feature selection using the Spider Monkey Optimization algorithm, which aims to minimize the number of features in cancer data.
2. The proposed method also includes the classification of cancer data using a subset of gene features obtained from the feature selection process.
3. Experimental results show that the proposed method outperforms existing techniques in terms of both the minimum number of features and maximum classification accuracy.
The article titled "Microarray Cancer Gene Feature Selection Using Spider Monkey Optimization Algorithm and Cancer Classification using SVM" discusses a new approach for cancer gene feature selection and classification using a swarm intelligence technique called the Spider Monkey Optimization algorithm. The article claims that this method outperforms all other existing techniques in terms of the minimum number of features and maximum classification accuracy.
One potential bias in this article is the lack of discussion about the limitations or potential risks associated with using the Spider Monkey Optimization algorithm. While the article mentions that it outperforms other techniques, it does not provide any evidence or analysis to support this claim. Additionally, there is no mention of any potential drawbacks or challenges that may arise when using this algorithm.
Another bias in this article is the focus on promoting the proposed method as superior to existing techniques without providing a balanced view of other approaches. The article only briefly mentions other methods such as genetic algorithms and artificial bee colony algorithms, but does not provide a comprehensive comparison or evaluation of these methods.
Furthermore, the article lacks detailed information about the datasets used for experimentation and validation. It does not provide any information about the size or characteristics of these datasets, which makes it difficult to assess the generalizability of the results.
Additionally, there is a lack of discussion about how the selected gene features are relevant to cancer classification. The article does not provide any explanation or analysis regarding why these specific genes were chosen and how they contribute to accurate classification.
Overall, this article presents an interesting approach for cancer gene feature selection and classification, but it lacks critical analysis and evidence to support its claims. There are biases towards promoting the proposed method without considering potential limitations or alternative approaches. More comprehensive research and analysis are needed to validate the effectiveness and generalizability of this method.