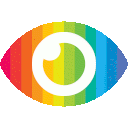
1. The proposed method for runway detection is based on airborne multi-sensors data fusion and works in a coarse-to-fine hierarchical architecture.
2. At the coarse layer, a vision projection model is built using airborne navigation data and forward-looking sensing images to extract a runway region of interest (ROI) from the image.
3. At the fine layer, EDLines, a real-time line segments detector, is used to extract straight line segments from the ROI, which are then linked into two long runway lines. Unique runway features are used to recognize the airport runway.
The article titled "Real-time Accurate Runway Detection based on Airborne Multi-sensors Fusion" presents a novel method for runway detection using airborne multi-sensor data fusion. The authors argue that existing methods for runway detection based on computer vision techniques are too complicated and time-consuming for real-time airborne applications.
One potential bias in this article is the lack of discussion or comparison with other existing methods for runway detection. The authors briefly mention that existing algorithms are complicated and time-consuming, but they do not provide any evidence or examples to support this claim. Without a thorough analysis of existing methods, it is difficult to determine whether the proposed method is truly superior.
Additionally, the article does not provide enough information about the dataset used for testing the proposed method. The authors mention that they tested their method on an image dataset provided by a flight simulation system, but they do not provide any details about the size or diversity of the dataset. This lack of information makes it difficult to assess the generalizability and reliability of the experimental results.
Furthermore, there is no discussion of potential risks or limitations associated with the proposed method. For example, it would be important to consider how well the method performs under different weather conditions or lighting conditions. Without addressing these potential risks, it is unclear whether the proposed method would be suitable for real-world applications.
The article also lacks a comprehensive analysis of alternative approaches to runway detection. While some related work is mentioned in passing, such as deep convolutional neural networks (CNNs) and line segment classification methods, there is no detailed comparison or evaluation of these approaches. This omission limits the reader's understanding of how the proposed method compares to other state-of-the-art techniques.
In terms of promotional content, it should be noted that this article was published on ResearchGate, which is a platform where researchers can share their work and collaborate with others in their field. Therefore, it is possible that this article may have been written with the intention of promoting the authors' research and attracting attention from other researchers.
Overall, this article presents a novel method for runway detection based on airborne multi-sensor data fusion. However, it lacks a thorough analysis of existing methods, does not provide enough information about the dataset used for testing, and fails to address potential risks or limitations. Additionally, there is a lack of comparison with alternative approaches to runway detection. These shortcomings limit the reader's ability to fully evaluate the proposed method and its potential contributions to the field.