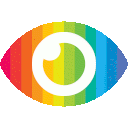
1. The study proposes a method to improve medical concept embeddings by incorporating hierarchical information from medical codes into the embedding formulation.
2. The Word2Vec models that included hierarchical data outperformed ordinary Word2Vec models on tasks comparing clusters of codes, achieving higher normalized mutual information with canonical labels.
3. Including hierarchical embedding data improved classification performance in 96.2% of cases, and co-training embeddings improved classification performance in 66.7% of cases, outperforming competitive benchmarks.
The article titled "Exploiting hierarchy in medical concept embedding" discusses the construction and release of medical concept embeddings for codes following the ICD-10 coding standard. The authors aim to incorporate hierarchical information from medical codes into the embedding formulation to improve model performance.
One potential bias in this article is the focus on positive results and the omission of any negative findings or limitations. The authors claim that their Word2Vec models with hierarchical data outperformed ordinary Word2Vec alternatives, improved classification performance, and significantly outperformed other pretrained vectors. However, they do not provide any evidence or discussion of potential drawbacks or limitations of their approach.
The article also lacks a comprehensive discussion of alternative methods or approaches to medical concept embedding. While they briefly mention Poincare embeddings as an alternative, they do not explore other existing techniques or compare their method to these alternatives. This narrow focus limits the reader's understanding of the broader landscape of medical concept embedding research.
Additionally, there is a lack of transparency regarding the dataset used for training and evaluation. The authors mention using data from a major integrated healthcare organization but do not provide details about the specific characteristics or representativeness of this dataset. Without this information, it is difficult to assess the generalizability of their findings.
Furthermore, there is no discussion of potential risks or ethical considerations associated with using medical concept embeddings in practice. Embeddings derived from patient health information have implications for privacy and security, and it would be important to address these concerns in an article discussing their use.
Overall, while the article presents some interesting findings regarding hierarchical data in medical concept embeddings, it suffers from biases towards positive results, lack of consideration for alternative approaches, limited transparency regarding data sources, and omission of potential risks and limitations. A more balanced and comprehensive analysis would strengthen the credibility and usefulness of this research.