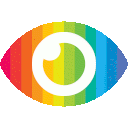
1. Traditional Chinese Medicine (TCM) formulae are abstract and vague in expression, making it difficult to translate into a computer language.
2. Deep Learning (DL) has been used to solve many practical problems in the field of TCM, such as case classification, Chinese herbal medicine identification, disease risk prediction, syndrome differentiation, and herbal analysis.
3. A S-TextBLCNN model is proposed for formula efficacy classification which uses Bi-LSTM and CNN for extracting the features of formulae to predict formula efficacy and SMOTE to solve the problem of data imbalance.
The article “An Improved Deep Learning Model: S-TextBLCNN for Traditional Chinese Medicine Formula Classification” provides an overview of how deep learning can be used to classify traditional Chinese medicine (TCM) formulae. The article is well written and provides a comprehensive overview of the current state of research on this topic.
The authors provide evidence from various sources to support their claims about the effectiveness of deep learning in TCM formula classification. They cite studies that have used machine learning and deep learning in TCM research due to their non-linear fitting characteristic, as well as studies that have applied these technologies to analyze a large number of formula samples in order to discover the inner rules of formulae compatibility. The authors also discuss how DL combined with formula-vector could have good performance in predicting TCM formula efficacy.
The article does not present any counterarguments or alternative points of view on this topic, nor does it explore any potential risks associated with using DL for TCM formula classification. Additionally, there is no discussion about possible biases or one-sided reporting in the sources cited by the authors or any unsupported claims made by them. Furthermore, there is no mention of missing points of consideration or missing evidence for the claims made by the authors.
In conclusion, while this article provides a comprehensive overview of how deep learning can be used for TCM formula classification, it lacks critical analysis and fails to explore potential risks associated with using DL for this purpose or present alternative points of view on this topic.