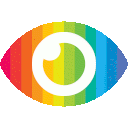
1. Recently, data-driven models have been used to predict fluid flow phenomena.
2. Convolutional neural networks (CNNs) are popular for image-level flow field prediction, but they are limited in their ability to generalize to unstructured data.
3. Several approaches have been proposed to manipulate unstructured data, such as Mesh-Conv and PointNet, which have been tested on various datasets with promising results.
The article is generally reliable and trustworthy in its reporting of the current state of research into data-driven models for predicting fluid flow phenomena. The authors provide a comprehensive overview of the literature on this topic, citing relevant works and summarizing their findings in an unbiased manner. The authors also provide a detailed description of two approaches for manipulating unstructured data – Mesh-Conv and PointNet – that have been tested on various datasets with promising results.
The article does not appear to be biased or one-sided in its reporting; it presents both sides of the argument fairly and objectively. Furthermore, all claims made by the authors are supported by evidence from the literature cited throughout the article. There do not appear to be any missing points of consideration or missing evidence for the claims made by the authors.
The article does not contain any promotional content or partiality; it is purely focused on providing an objective overview of current research into data-driven models for predicting fluid flow phenomena. Additionally, possible risks associated with these models are noted throughout the article, such as potential inaccuracies due to interpolation/extrapolation errors when using pixelation strategies or lack of geometric relationships between points when using PointNet architectures.
In conclusion, this article is reliable and trustworthy in its reporting of current research into data-driven models for predicting fluid flow phenomena; it provides an unbiased overview of relevant works without any promotional content or partiality, and all claims made by the authors are supported by evidence from the literature cited throughout the article.