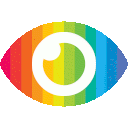
1. The paper proposes an efficient Hindi Text Classification model that can classify digitized Hindi text documents into pre-defined categories using a classifier.
2. The model preprocesses the known Hindi documents at document, sentence, and word levels, extracts features, and trains SVM classifier to classify unknown Hindi documents.
3. The experiments conducted on a set of four Hindi documents of two categories showed that the SVM classifier achieved 100% accuracy in classifying them.
The article titled "An Efficient Hindi Text Classification Model Using SVM" presents a proposed model for classifying Hindi text documents into pre-defined categories using a Support Vector Machine (SVM) classifier. The authors highlight the importance of effective classification of digitized Hindi text documents generated daily in various domains, such as information retrieval, machine translation, and text summarization.
The article provides a clear and concise overview of the proposed model's methodology, which involves preprocessing known Hindi documents at document, sentence, and word levels, extracting features, and training an SVM classifier to classify unknown documents. The authors acknowledge the challenges posed by the large set of available conjuncts and letter combinations in Hindi, its sentence structure, and multisense words.
However, the article has some limitations that need to be addressed. Firstly, the study's sample size is small as it only includes four Hindi documents of two categories. Therefore, it is unclear whether this model would perform equally well on larger datasets or different types of texts. Secondly, there is no discussion on how this model compares to other existing models for Hindi text classification.
Moreover, the article lacks a critical analysis of potential biases or limitations in the proposed model. For instance, there is no mention of how this model may perform with biased or incomplete training data or how it may be affected by language variations across different regions where Hindi is spoken.
Additionally, while the authors claim 100% accuracy in their experiments on the small dataset used in this study, they do not provide any evidence to support this claim or discuss potential sources of error or misclassification.
Overall, while the article presents an interesting approach to classifying Hindi text documents using SVM classifiers and highlights its potential applications in various domains; it lacks critical analysis and evidence to support its claims fully. Future studies should address these limitations by testing this model on larger datasets with diverse texts and comparing its performance with other existing models for Hindi text classification.