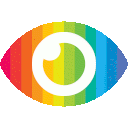
1. Existing Graph Neural Networks (GNNs) are not suitable for real-world situations where a few classes dominate other classes and the node degree distribution is imbalanced.
2. A novel framework, Long-Tail Experts for Graphs (LTE4G), is proposed to jointly consider class long-tailedness and degree long-tailedness for node classification.
3. LTE4G outperforms existing state-of-the-art methods in node classification on both manual and natural imbalanced graphs.
The article provides a detailed overview of the proposed Long-Tail Experts for Graphs (LTE4G) framework, which is designed to address the issue of class and degree long-tailedness in graph neural networks (GNNs). The article presents evidence that LTE4G outperforms existing state-of-the-art methods in node classification on both manual and natural imbalanced graphs. The article also provides a link to the source code of LTE4G, which allows readers to verify its claims.
The article does not present any counterarguments or alternative approaches to addressing class and degree long-tailedness in GNNs, nor does it discuss any potential risks associated with using LTE4G. Additionally, there is no discussion of how the results of this research could be applied in practice or what implications they may have for future research. Furthermore, there is no mention of any potential biases or sources of bias that may have influenced the results presented in the paper.
In conclusion, while this article provides an interesting overview of LTE4G and its potential applications, it lacks some important points of consideration such as counterarguments, potential risks associated with using LTE4G, and sources of bias that may have influenced the results presented in the paper.