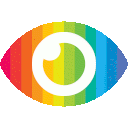
1. Frog-GNN is a multi-perspective aggregation based graph neural network for few-shot text classification that focuses on all query-support pairs without information loss.
2. The model combines the benefits of pre-trained language models and graph neural networks with a multi-perspective aggregation strategy to retain all the features of sample pairs and avoid information loss effectively.
3. Frog-GNN outperforms existing few-shot models in both text classification and relation classification, and ablation experiments show the effectiveness of its multi-perspective aggregation.
The article titled "Frog-GNN: Multi-perspective aggregation based graph neural network for few-shot text classification" presents a new approach to few-shot text classification using a multi-perspective aggregation based graph neural network. The authors argue that previous approaches, such as Prototypical Networks, have limitations in their prototype aggregation progress, which ignores much useful information of support set and discrepancy between samples from different classes. In contrast, the proposed model focuses on all query-support pairs without information loss.
The article provides a detailed description of the proposed Frog-GNN model and its advantages over existing models. The authors claim that their model combines the benefits of pre-trained language models and graph neural networks with a multi-perspective aggregation strategy dedicated to text classification. They also argue that their model is fit to process complex natural language and can retain all the features of sample pairs while avoiding information loss effectively.
While the article provides a thorough explanation of the proposed model and its advantages, it lacks discussion on potential biases or limitations of the approach. For example, it is unclear how well the model performs on datasets with different characteristics or how sensitive it is to noisy or irrelevant information in the support set. Additionally, there is no discussion on potential risks associated with using machine learning models for text classification, such as perpetuating biases or misclassifying sensitive information.
Furthermore, the article does not provide an in-depth analysis of counterarguments or alternative approaches to few-shot text classification. While the authors briefly mention previous works based on metric learning and optimization-based methods, they do not discuss their limitations or potential advantages over their proposed approach.
Overall, while the article presents an interesting new approach to few-shot text classification using a multi-perspective aggregation based graph neural network, it could benefit from more critical analysis and discussion on potential biases and limitations of the approach.