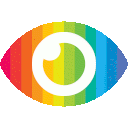
1. The use of single-cell RNA sequencing (scnRNA-seq) technologies can help characterize the composition of tissues and reveal cells that influence key functions in both healthy and disease tissues.
2. Computational deconvolution methods that infer the composition of bulk-profiled samples using scnRNA-seq-characterized cell types can broaden scnRNA-seq applications, but their effectiveness remains controversial.
3. The proposed method, Single-cell RNA Quantity Informed Deconvolution (SQUID), which combines RNA-seq transformation and dampened weighted least-squares deconvolution approaches, consistently outperformed other methods in predicting the composition of cell mixtures and tissue samples.
The article titled "Effective methods for bulk RNA-seq deconvolution using scnRNA-seq transcriptomes" published in Genome Biology discusses the challenges and potential solutions for using single-cell RNA sequencing (scnRNA-seq) data to infer the composition of bulk RNA-seq samples. The authors evaluate different deconvolution methods and propose a new method called Single-cell RNA Quantity Informed Deconvolution (SQUID) that combines RNA-seq transformation and weighted least-squares deconvolution approaches.
Overall, the article provides a comprehensive analysis of the effectiveness of different deconvolution methods and highlights the importance of accurate data preprocessing and method selection. The authors also demonstrate the potential benefits of using concurrent RNA-seq and scnRNA-seq profiles to improve deconvolution accuracy.
However, there are several potential biases and limitations in the article that should be considered. Firstly, the evaluation of deconvolution methods is based on datasets with either known or scnRNA-seq-estimated compositions. While this provides a benchmark for comparison, it may not fully represent real-world scenarios where the true composition is unknown. Additionally, the article focuses primarily on cancer research applications, specifically pediatric acute myeloid leukemia (AML) and neuroblastoma. This narrow focus limits the generalizability of the findings to other diseases or tissue types.
Furthermore, while SQUID consistently outperforms other methods in predicting cell-type abundance, it is unclear how well it performs compared to other existing deconvolution methods that were not included in this study. The authors claim that SQUID's accuracy improvement is vital for its potential applications in diagnostic protocols for cancer patients, but more evidence is needed to support this claim.
Another limitation is that the article does not thoroughly explore potential risks or limitations associated with using scnRNA-seq data for deconvolution. For example, there may be biases introduced during sample collection, processing, and storage that could affect the accuracy of deconvolution methods. Additionally, the high cost and stringent sample-collection requirements of scnRNA-seq assays are mentioned as challenges, but potential solutions or alternatives are not discussed.
In terms of reporting bias, the article primarily focuses on the positive aspects and potential benefits of using scnRNA-seq data for deconvolution. While this is understandable given the goal of the study, it would have been beneficial to also discuss any limitations or drawbacks associated with this approach.
Overall, while the article provides valuable insights into the effectiveness of different deconvolution methods and proposes a new method that shows promise, there are several biases and limitations that should be considered. Further research is needed to validate the findings in different disease contexts and to address potential risks and limitations associated with using scnRNA-seq data for deconvolution.