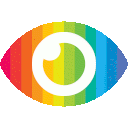
1. SCENIC is a workflow that uses three R/bioconductor packages (GENIE3, RcisTarget, and AUCell) to infer gene regulatory networks and cluster single cells based on their activity.
2. GENIE3 is a method for inferring gene regulatory networks from gene expression data using random forest models, while GRNBoost uses gradient-boosting machines to infer regulators for each target gene.
3. RcisTarget identifies enriched TF-binding motifs and candidate transcription factors for a gene list, while AUCell calculates the enrichment of regulons in single-cell RNA-seq data to identify cells with active gene regulatory networks.
The article titled "SCENIC: single-cell regulatory network inference and clustering" provides an overview of the SCENIC workflow, which is a method for inferring gene regulatory networks and clustering single cells based on their gene expression data. While the article provides detailed information about the different components of the workflow and the tools used, there are several aspects that need to be critically analyzed.
One potential bias in the article is the lack of discussion about the limitations of the SCENIC workflow. The authors mention that they have applied SCENIC to multiple datasets and obtained reliable results, but they do not provide any information about cases where SCENIC may not work well or situations where it may produce inaccurate results. This lack of discussion about potential limitations could give readers a false sense of confidence in the method.
Another issue with the article is its focus on promoting the SCENIC workflow without providing a balanced view of other existing methods for inferring gene regulatory networks and clustering single cells. While the authors briefly mention other methods such as i-cisTarget and iRegulon, they do not provide a comprehensive comparison of these methods with SCENIC. This one-sided reporting could lead readers to believe that SCENIC is superior to other methods without considering alternative approaches.
Additionally, there are unsupported claims in the article, such as stating that GENIE3 is the top-performing method for network inference in certain challenges without providing evidence or references to support this claim. The authors also claim that GRNBoost outperforms GENIE3 in terms of scalability but do not provide any benchmarking results or comparisons between these two methods.
Furthermore, there are missing points of consideration in the article. For example, while the authors mention that within-sample normalizations may induce artificial covariation and recommend avoiding them for coexpression analysis, they do not discuss alternative normalization methods or their potential impact on network inference results. This omission limits readers' understanding of the potential effects of different normalization approaches on the SCENIC workflow.
The article also lacks exploration of counterarguments or alternative perspectives. The authors present SCENIC as a comprehensive and effective method for gene regulatory network inference and clustering, but they do not discuss any potential criticisms or limitations that other researchers may have raised about the method. This lack of critical analysis could give readers a biased view of the strengths and weaknesses of SCENIC.
In terms of promotional content, the article heavily promotes the SCENIC workflow and its associated tools without providing a balanced view of their capabilities or limitations. The authors provide links to the tools and tutorials, which may give readers the impression that they are endorsing these tools without considering alternative options.
Overall, while the article provides detailed information about the SCENIC workflow and its components, it has several biases and shortcomings that need to be critically analyzed. These include one-sided reporting, unsupported claims, missing points of consideration, unexplored counterarguments, promotional content, and lack of discussion about limitations. A more balanced and comprehensive analysis would provide readers with a more accurate understanding of the strengths and weaknesses of the SCENIC workflow.