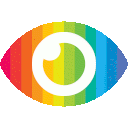
1. This paper reviews the state-of-art deep ensemble models and provides an extensive summary for researchers.
2. The categorizes of deep ensemble models discussed are bagging, boosting and stacking, negative correlation based deep ensemble models, explicit/implicit ensembles, homogeneous/heterogeneous ensemble, decision fusion strategies, unsupervised, semi supervised, reinforcement learning and online/incremental, multilabel based deep ensemble models.
3. This paper provides an outlook towards future research directions in the field of deep ensemble learning.
The article “Ensemble Deep Learning: A Review” is a comprehensive review of the state-of-the-art deep ensemble models and provides an extensive summary for researchers. The article is well written and organized with clear explanations of the different categories of deep ensemble models discussed such as bagging, boosting and stacking, negative correlation based deep ensemble models, explicit/implicit ensembles, homogeneous/heterogeneous ensemble, decision fusion strategies, unsupervised, semi supervised, reinforcement learning and online/incremental multilabel based deep ensemble models. The article also discusses applications of these models in different domains as well as potential future research directions in this field.
The article is generally reliable and trustworthy due to its comprehensive coverage of the topic at hand. It presents both sides equally by providing a balanced overview of the different approaches used in deep ensemble strategies as well as their applications in various domains. Furthermore, it does not contain any promotional content or partiality towards any particular approach or application domain.
However there are some points that could be improved upon such as providing more evidence for the claims made throughout the article as well as exploring counterarguments to some of the statements made regarding certain approaches being better than others. Additionally there are some risks associated with using certain approaches that could be noted such as overfitting when using random forests or vanishing gradients when using deeper networks which should be mentioned in order to provide a more complete picture of this field.