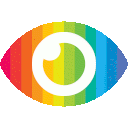
1. The article proposes a method based on Deep Reinforcement Learning (DRL) for multi-agent formation control and obstacle avoidance.
2. The method uses bearing-based and angle-based reward functions to maintain the shape of the formation while navigating around obstacles.
3. The approach is compared with a different method using an angle-based reward function, demonstrating its effectiveness in maintaining formation shape with varying formation size.
The article titled "Proximal policy optimization for formation navigation and obstacle avoidance" discusses the use of deep reinforcement learning (DRL) for multi-agent formation control and obstacle avoidance. The authors highlight the challenges of maintaining the shape of a formation while maneuvering around obstacles and propose a DRL-based method to address this problem.
Overall, the article provides a comprehensive overview of related works in the field and presents the contributions of their proposed approach. However, there are several aspects that need to be critically analyzed.
Firstly, the article lacks a clear discussion on potential biases and their sources. While it mentions traditional control methods as established approaches, it does not provide a balanced comparison between these methods and DRL. This omission could suggest a bias towards promoting DRL as the superior solution without considering potential limitations or drawbacks.
Additionally, the article makes unsupported claims about the advantages of DRL over conventional control methods. It states that DRL offers near optimal control without model specification and generalizability to different operating conditions, unmodeled dynamics, and constraints. However, no evidence or references are provided to support these claims. Without empirical data or comparative studies, it is difficult to assess the validity of these assertions.
Furthermore, there is limited discussion on potential risks or limitations associated with using DRL for formation control and obstacle avoidance. While the authors mention that their approach allows for flexible maintenance of formation shape, they do not address potential challenges or failure modes that may arise in real-world scenarios. This lack of consideration for possible risks undermines the credibility of their proposed method.
The article also lacks exploration of counterarguments or alternative perspectives. It primarily focuses on promoting their own approach without acknowledging potential criticisms or limitations. A more balanced analysis would have included discussions on alternative methods or approaches that may have been considered but ultimately rejected in favor of DRL.
In terms of missing evidence, the article does not provide any simulation results or empirical data to support their claims. While they mention that simulation results are included, these results are not presented in the article itself. Without access to this data, it is difficult to evaluate the effectiveness or performance of their proposed method.
Lastly, the article contains some promotional content by highlighting the advantages of their approach over previous works without providing a comprehensive analysis of the limitations or drawbacks of those previous approaches. This one-sided reporting could create a biased view and mislead readers into believing that DRL is the only viable solution for formation control and obstacle avoidance.
In conclusion, while the article provides an overview of the proposed DRL-based method for multi-agent formation control and obstacle avoidance, it lacks critical analysis and balanced reporting. The absence of evidence, unsupported claims, missing counterarguments, and promotional content undermine the credibility and objectivity of the article. Further research and empirical studies are needed to validate the effectiveness and limitations of DRL in this context.