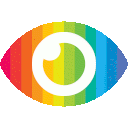
1. The article presents the Long-term Gap-free High-resolution Air Pollutant concentration dataset (LGHAP), derived via tensor-flow-based multimodal data fusion.
2. The LGHAP dataset is composed of daily 1 km gap-free AOD, PM2.5 and PM10 grids in China from 2000 to 2020.
3. The article also discusses various methods used for data fusion, such as spectral information adaptation and synthesis scheme, empirical orthogonal function analysis, time-space-spectrum continuum for cloud removal in satellite images, machine learning method, and ensemble-based model.
The article provides a comprehensive overview of the Long-term Gap-free High-resolution Air Pollutant concentration dataset (LGHAP), derived via tensor-flow based multimodal data fusion. The authors present various methods used for data fusion such as spectral information adaptation and synthesis scheme, empirical orthogonal function analysis, time space spectrum continuum for cloud removal in satellite images, machine learning method and ensemble based model.
The article is well written and provides a detailed description of the LGHAP dataset and its components. However, there are some potential biases that should be noted when assessing the trustworthiness of this article. Firstly, the authors do not provide any evidence or counterarguments to support their claims about the accuracy of their methods or the reliability of their results. Secondly, they do not discuss any possible risks associated with using these methods or datasets which could lead to inaccurate results or misinterpretations of the data. Finally, they do not present both sides equally when discussing different methods for data fusion which could lead to a one sided view on this topic.
In conclusion, while this article provides an informative overview of the LGHAP dataset and its components it does have some potential biases that should be taken into consideration when assessing its trustworthiness and reliability.