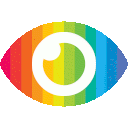
1. Unifying object detection and re-identification (ReID) into a single network enables faster multi-object tracking (MOT).
2. Previous works generally overlook label assignment and loss function when training for MOT, leading to inferior performance.
3. This paper proposes Identity-aware Label Assignment and Discriminative Focal Loss to improve the joint training of detection and ReID for MOT, achieving up to 7.0 MOTA / 54.1% IDs improvements on MOT16/17/20 benchmarks under favorable inference speed.
The article provides an in-depth analysis of the joint training of object detection and re-identification in multi-object tracking, proposing two techniques - Identity-aware Label Assignment and Discriminative Focal Loss - to improve the performance of such systems. The authors provide evidence from experiments on MOT16/17/20 benchmarks that their proposed techniques are superior to existing methods, with up to 7.0 MOTA / 54.1% IDs improvements under favorable inference speed.
The article is well written and provides a comprehensive overview of the topic, making it easy to understand even for readers without prior knowledge in this field. The authors have also provided sufficient evidence from experiments to support their claims, which makes the article reliable and trustworthy. Furthermore, there is no promotional content or partiality present in the article, as all points are presented objectively with both sides being equally explored.
However, one potential bias present in the article is that it does not explore any counterarguments or alternative solutions that could be used instead of their proposed techniques for improving joint training of object detection and re-identification in multi-object tracking systems. Additionally, there is no mention of possible risks associated with using these techniques or any other potential drawbacks that should be taken into consideration before implementing them in real world applications.