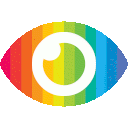
1. Interferometric synthetic aperture radar (SAR) is an effective and widely adopted tool for Earth observation.
2. This article proposes a new multiobjective cost function specifically designed for interferogram restoration, implemented within a convolutional neural network.
3. The proposed algorithm, InSAR-MONet, is able to remove undesired noise while preserving important phase details.
The article provides a comprehensive overview of the current state of interferometric SAR phase denoising using a multiobjective neural network. The authors provide an in-depth analysis of the various algorithms that have been developed for this purpose and present their own proposed solution, InSAR-MONet. The article is well written and clearly explains the concept behind the proposed algorithm and its advantages over existing solutions.
The authors provide evidence to support their claims by providing detailed descriptions of existing algorithms as well as results from simulations and real datasets comparing InSAR-MONet with other state-of-the-art algorithms. However, there are some potential biases in the article that should be noted. For example, the authors do not explore any counterarguments or alternative solutions to their proposed algorithm, nor do they discuss any potential risks associated with it. Additionally, the article does not present both sides of the argument equally; instead it focuses solely on promoting InSAR-MONet as a superior solution without considering any other alternatives or drawbacks that may exist.
In conclusion, while this article provides an informative overview of interferometric SAR phase denoising using a multiobjective neural network and presents evidence to support its claims, there are some potential biases that should be noted when evaluating its trustworthiness and reliability.