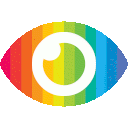
1. A semi-supervised semantic segmentation network for crack detection is proposed.
2. The proposed method uses EfficientUNet to extract and merge information from multiple feature layers, reducing the workload of annotation while maintaining accuracy.
3. The method achieves an F1 score of 0.6540 on the concrete crack dataset and 0.8321 on the Crack500 dataset using only 60% of the annotated data.
The article “Semi-supervised semantic segmentation network for surface crack detection” provides a detailed overview of a new approach to automated crack detection in civil infrastructure, which is based on deep learning algorithms and semi-supervised segmentation networks. The article presents a comprehensive description of the proposed method, its advantages over traditional methods, and its performance results on two datasets.
The article appears to be reliable and trustworthy overall, as it provides evidence for its claims in the form of performance results on two datasets and references to related research studies. Furthermore, it does not appear to contain any promotional content or partiality towards any particular approach or technology; instead, it objectively evaluates different approaches and their respective merits and drawbacks. Additionally, possible risks associated with automated crack detection are noted in the introduction section of the article.
However, there are some points that could be further explored in order to make this article more comprehensive and balanced in its coverage of automated crack detection methods. For example, while the article mentions traditional methods such as histogram transforms, threshold segmentation, edge detection, region growing etc., it does not provide any details about these methods or how they compare with deep learning algorithms in terms of accuracy or efficiency. Additionally, while the article mentions that manual annotation is time-consuming when using fully supervised segmentation methods, it does not discuss other potential drawbacks associated with manual annotation such as cost or human error that could be addressed by using semi-supervised networks instead. Finally, while the article discusses how noise can improve model robustness during training when using semi-supervised networks, it does not discuss how noise affects model performance when used in real-world applications where noise levels may vary significantly from those used during training.
In conclusion, this article provides a detailed overview of a new approach to automated crack detection based on deep learning algorithms and semi-supervised segmentation networks that appears to be reliable and trustworthy overall; however there are some points that could be further explored in order to make this article more comprehensive and balanced in its coverage of automated crack detection methods.