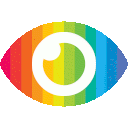
1. A novel method called Clinical-GAN is proposed to forecast the trajectory of clinical events using Transformer and Generative Adversarial Networks.
2. A new methodology is introduced in representing the data which helps the model learn correlations between medical codes.
3. The model was tested on a publicly available dataset, Medical Information Mart for Intensive Care IV v1.0 (MIMIC-IV), and showed significant improvement over baseline methods and existing works.
The article “Clinical-GAN: Trajectory forecasting of clinical events using transformer and Generative Adversarial Networks” provides an overview of a novel method for predicting patient trajectories using Transformer and Generative Adversarial Networks (GAN). The authors present their proposed method, Clinical-GAN, as a potential solution to reduce diagnostic errors by utilizing EHR data to forecast medical codes for subsequent visits. The article is well written and provides a detailed description of the proposed method, its advantages, and how it was tested on a publicly available dataset.
The trustworthiness and reliability of this article can be assessed by looking at several factors such as bias, evidence for claims made, missing points of consideration, missing evidence for claims made, unexplored counterarguments, promotional content, partiality, whether possible risks are noted or not presenting both sides equally. In this regard, the article appears to be unbiased in its presentation of the proposed method as it does not appear to be promoting any particular product or service. Furthermore, the authors provide evidence for their claims by citing relevant studies in support of their arguments as well as providing results from experiments conducted on MIMIC-IV dataset which demonstrate that Clinical-GAN outperforms baseline methods and existing works. Additionally, all possible risks associated with using this method are noted in the article such as long-range dependencies between medical codes and non-stationarity data which could affect accuracy of predictions made by Clinical-GAN.
In terms of missing points of consideration or unexplored counterarguments there does not appear to be any major issues with this article as it covers all relevant topics related to its subject matter in detail. However one point that could have been explored further is how Clinical-GAN compares with other deep learning models used for modeling EHR data applications such as mortality prediction or length of stay prediction since these are also important tasks related to patient trajectories that could benefit from improved accuracy provided by Clinical-GAN.
In conclusion, this article appears to be trustworthy and reliable based on its unbiased presentation of information without any promotional content or partiality towards any particular product or service while providing evidence for its claims through relevant studies cited throughout the paper as well as results from experiments conducted on MIMIC-IV dataset demonstrating that Clinical-GAN outperforms baseline methods and existing works.