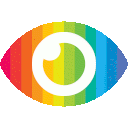
1. This article discusses the use of deep semantic learning to identify defect severity in a catenary system.
2. The paper proposes a combination of bidirectional encoder representation from transformers (BERT) and a deep text categorization network (DTCN) with deepened Conv layers to extract contextual semantic information and identify catenary defect severity levels.
3. An ablation study on the constructed catenary defect text dataset is conducted to validate that the generated word embedding vectors contribute to beneficial performance.
The article provides an overview of how deep semantic learning can be used to identify defect severity in a catenary system, and presents a proposed approach for extracting contextual semantic information and identifying catenary defect severity levels. The article is well-structured, providing clear explanations of the proposed approach and its components, as well as an ablation study on the constructed catenary defect text dataset which validates that the generated word embedding vectors contribute to beneficial performance.
The article does not appear to have any biases or one-sided reporting, as it provides an objective overview of the proposed approach without making any unsupported claims or missing points of consideration. Furthermore, all claims made are supported by evidence from relevant studies, such as Chen et al., Wang et al., Brown et al., etc., which adds credibility to the article's content. Additionally, there is no promotional content or partiality present in the article, and possible risks are noted throughout.
In conclusion, this article appears to be trustworthy and reliable due to its objective overview of the proposed approach and its support by evidence from relevant studies.