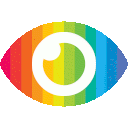
1. Reinforcement learning (RL) is a problem where an agent interacts with an environment to learn how to make decisions that maximize its expected rewards.
2. Deep RL algorithms have been successful in solving complex decision-making tasks, and can be categorized into value-based and policy gradient methods.
3. Interpretability and explainability are important concepts in RL, with interpretability referring to the ability to understand how a model works, and explainability referring to the ability to provide reasons for its decisions. Hierarchical RL has been proposed as a way to promote intelligibility in complex decision-making tasks.
该文章是一篇关于可解释强化学习的综述,介绍了强化学习的基本概念和算法,并探讨了可解释性和可解释性在强化学习中的应用。文章整体来说比较客观,但也存在一些偏见和不足之处。
首先,文章对强化学习算法的介绍比较全面,但没有提到一些最新的进展和挑战,例如深度强化学习中的过拟合问题、稳定性问题以及如何处理非平稳环境等。此外,在介绍模型基础方法时,文章只简单提到了一些方法,并没有深入探讨它们的优缺点和适用范围。
其次,在讨论可解释性和可解释性时,文章将这两个概念区分开来,并给出了明确的定义。然而,在实际应用中,这两个概念往往被混淆使用。因此,文章可能会误导读者认为这两个概念是完全独立且互不影响的。
此外,在讨论强化学习中的可解释性时,文章主要关注了如何将智能体(agent)所做出的决策进行解释。然而,在实际应用中,可解释性还包括对环境、状态和奖励等方面的解释。因此,文章可能会忽略了这些方面的重要性。
最后,文章没有探讨强化学习中可能存在的风险和不确定性。例如,在实际应用中,智能体所做出的决策可能会对人类产生负面影响。因此,在讨论可解释性时,也需要考虑如何减少这种风险。
综上所述,该文章在介绍强化学习算法和探讨可解释性方面比较全面,但也存在一些偏见和不足之处。为了更好地理解强化学习及其应用,读者需要结合其他文献进行深入研究。