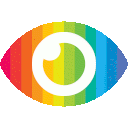
1. Automatic Curriculum Learning (ACL) is a family of mechanisms that adapt the distribution of training data by adjusting the selection of learning situations to the capabilities of learning agents in Deep Reinforcement Learning (DRL).
2. ACL mechanisms can act on many aspects of learning problems, such as optimizing domain randomization for Sim2Real transfer, organizing task presentations in multi-task robotic settings, and ordering sequences of opponents in multi-agent scenarios.
3. ACL has been used for diverse purposes, including improving performance on a restricted task set, solving hard tasks, and encouraging generalization or solving sparse reward problems.
该文章提供了关于自动课程学习(ACL)在深度强化学习(DRL)中的应用的简要综述。然而,该文章存在一些偏见和不足之处。
首先,该文章没有探讨ACL可能带来的风险和负面影响。例如,在某些情况下,ACL可能会导致过度拟合或过度专业化,从而使代理人无法适应新的环境或任务。此外,ACL可能会导致代理人对特定类型的任务进行过度优化,而忽略其他类型的任务。
其次,该文章没有充分考虑到ACL机制与其他领域之间的联系。例如,在连续学习中使用的机制可以与ACL结合使用以提高代理人对未知环境变化的鲁棒性。
此外,该文章没有提供足够的证据来支持其所述观点。虽然该文章提到了一些实验结果和案例研究,但它没有详细说明这些结果是如何得出的,并且没有提供足够的数据来支持其结论。
最后,该文章似乎偏袒ACL机制,并未探讨其局限性和缺陷。例如,在某些情况下,ACL可能会导致代理人无法适应新环境或任务,并且可能会导致代理人对特定类型的任务进行过度优化,而忽略其他类型的任务。
综上所述,该文章提供了有关ACL在DRL中应用的简要综述,但存在一些偏见和不足之处。未来的研究应更加全面地探讨ACL机制的潜在风险和负面影响,并将其与其他领域的机制结合使用以提高代理人的鲁棒性。此外,未来的研究应提供更多证据来支持其结论,并探讨ACL机制的局限性和缺陷。