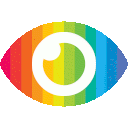
1. This article presents a novel unsupervised feature selection method called OCLSP, which combines feature selection, matrix factorization, and adaptive graph learning into a unified framework.
2. OCLSP is distinct from existing methods in that it decomposes the projected data points by orthogonal basis clustering to improve the quality of the projection matrix (which is also the feature selection matrix).
3. The proposed method also introduces an additional constraint on the target matrix T to ensure that it has the capability to discriminate projected clusters.
The article “OCLSP--21’TNNLS--Unsupervised Feature Selection Via Orthogonal Basis Clustering and Local Structure Preserving” provides a comprehensive overview of a novel unsupervised feature selection method called OCLSP. The authors present their approach in detail and provide evidence for its effectiveness compared to existing methods.
The article is well-written and provides clear explanations of the proposed approach as well as comparisons with existing methods. The authors have done an excellent job of presenting their research in an organized manner, making it easy for readers to understand their ideas and results.
However, there are some potential biases in the article that should be noted. For example, while the authors do mention some existing methods such as UDFS, NDFS, RUFS, NMF, SOCFS etc., they do not provide any detailed comparison between these methods and OCLSP or discuss how they differ from each other. Additionally, while the authors do mention some potential risks associated with their approach (e.g., overfitting), they do not provide any detailed discussion on how these risks can be mitigated or avoided altogether.
In conclusion, this article provides a comprehensive overview of a novel unsupervised feature selection method called OCLSP and presents evidence for its effectiveness compared to existing methods. However, there are some potential biases in the article that should be noted such as lack of detailed comparison between existing methods and OCLSP as well as lack of discussion on how potential risks associated with their approach can be mitigated or avoided altogether.