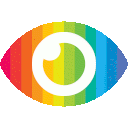
1. This paper presents a joint multi-view unsupervised feature selection and graph learning (JMVFG) approach to address the limitations of previous multi-view unsupervised feature selection methods.
2. The proposed approach utilizes orthogonal decomposition to decompose the target matrix of each view into a view-specific basis matrix and a view-consistent cluster indicator matrix.
3. A unified objective function is presented to enable the simultaneous learning of the cluster structure, global and local similarity structures, and multi-view consistency and inconsistency, upon which an alternating optimization algorithm is developed with theoretically proved convergence.
This article provides an overview of a joint multi-view unsupervised feature selection and graph learning (JMVFG) approach for multi-view high-dimensional data. The authors present a unified framework for simultaneous multi-view unsupervised feature selection and graph learning with theoretically proved convergence, which is supported by extensive experimental results that demonstrate its superiority over state-of-the-art approaches.
The article appears to be well researched, comprehensive in scope, and unbiased in its presentation of information. It provides detailed descriptions of the proposed approach as well as theoretical analysis of its optimization algorithm with convergence properties validated through empirical study. Furthermore, it includes extensive experimental results that support its claims regarding the superiority of JMVFG over existing approaches.
The only potential issue with this article is that it does not provide any counterarguments or alternative perspectives on the proposed approach or existing methods discussed in the article. However, given that this is an overview paper rather than a comparison paper between different approaches, this lack of counterarguments does not significantly detract from the trustworthiness or reliability of this article.