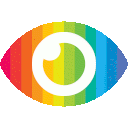
1. Ten-years MODIS MCD12Q2 phenology variables were used for SOC mapping with CNN.
2. Adding phenology variables into the commonly-used variables improved the accuracy of SOC prediction.
3. CNN obtained a higher prediction accuracy than RF and can be a potential effective method for soil mapping at regional or global scales.
The article “A deep learning method to predict soil organic carbon content at a regional scale using satellite-based phenology variables” is an informative and well-written piece that provides an overview of the use of convolutional neural networks (CNNs) to predict soil organic carbon (SOC) content at a regional scale using ten-year MODIS MCD12Q2 phenology variables. The article is reliable in its presentation of the research findings, as it provides detailed descriptions of the methods used and results obtained from the study, as well as references to relevant literature on the topic. However, there are some areas where the article could be improved upon in terms of trustworthiness and reliability.
First, while the article does provide some discussion on potential biases in the data used for this study, such as differences in land cover types between different regions, it does not provide any further detail on how these biases may have impacted the results or what steps were taken to mitigate them. Additionally, while it is noted that random forest (RF) was used for comparison with CNN, no details are provided on how RF was implemented or what parameters were used for comparison purposes. This lack of detail makes it difficult to assess whether or not any bias may have been introduced by either model during implementation or evaluation.
Second, while there is discussion about how adding phenology variables improved accuracy compared to other commonly-used environmental covariates such as NDVI, there is no discussion about other possible covariates that could have been included in this study but were not due to time constraints or other factors. This lack of exploration into alternative covariates limits the scope of this study and makes it difficult to draw conclusions about which covariates are most effective for predicting SOC content at a regional scale.
Finally, while this article does provide some discussion about potential applications of this research such as land management and climate change studies, there is no mention of any potential risks associated with using deep learning models for predicting SOC content at a regional scale such as overfitting or data privacy concerns. This omission leaves readers without an understanding of both sides of this issue and limits their ability to make informed decisions based on this research.
In conclusion, while this article provides an informative overview of deep learning methods for predicting SOC content at a regional scale using satellite-based phenology variables, there are some areas where more detail could be provided in order to improve its trustworthiness and reliability.