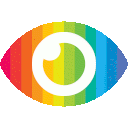
1. The article discusses the increasing rate of email spam and the need for a comprehensive system for spam classification based on natural language processing (NLP) and URL filtering.
2. Various machine learning algorithms are surveyed to create a high-performance and efficient model for text classification in spam emails.
3. The article also explores the integration of text classification and URL filtering to develop an add-on for Gmail that can effectively identify and filter out spam emails.
The article titled "E-Mail Spam Classification via Machine Learning and Natural Language Processing" discusses the importance of building a comprehensive system for spam classification in emails. It highlights the increasing rate of unsolicited bulk mail or spam and the need to address this issue using machine learning algorithms and natural language processing techniques.
One potential bias in the article is its focus on the negative aspects of spam emails, such as extracting confidential information, promoting adult content, and marketing/advertising products and services. While these are certainly valid concerns, it would have been beneficial to also mention the potential benefits of email marketing when used responsibly by legitimate businesses. Ignoring this aspect may create a one-sided view of email marketing as solely negative.
The article claims that various machine learning algorithms have been surveyed, but it does not provide any specific details about these algorithms or their evaluation criteria. This lack of information makes it difficult to assess the credibility and effectiveness of the proposed model for spam classification.
Additionally, the article mentions URL filtering as part of the approach but does not provide sufficient explanation or evidence for its effectiveness in spam classification. It would have been helpful to include examples or case studies demonstrating how URL filtering can enhance the accuracy of spam detection.
Furthermore, while the article acknowledges the importance of end-to-end encryption for protecting sensitive information in emails, it does not delve into any potential risks or limitations associated with encryption methods. A more comprehensive analysis would have considered both the benefits and challenges of implementing encryption in email communication.
The article also lacks exploration of counterarguments or alternative approaches to spam classification. It would have been valuable to discuss any existing limitations or criticisms of machine learning-based methods for spam detection and consider alternative solutions that could complement or improve upon these techniques.
In terms of promotional content, there is a mention at the end of integrating text classification and URL filtering to create an add-on on Gmail. This raises questions about whether this article is purely informative or if there is a commercial interest in promoting a specific product or service.
Overall, the article provides a general overview of the importance of spam classification in emails and mentions the use of machine learning and natural language processing techniques. However, it lacks specific details, evidence, and balanced analysis that would strengthen its credibility and provide a more comprehensive understanding of the topic.