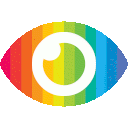
1. The article discusses the problem of spam comments on social media platforms and the need to automatically filter them.
2. The authors propose a method to identify different types of spam comments based on features such as discontinuous text, inadequate content, and unrelated comment topics.
3. The system they built uses natural language processing techniques and machine learning algorithms to detect spam comments, and its performance is evaluated using a benchmark dataset.
The article titled "Identification of spam comments using natural language processing techniques" discusses the problem of spam comments on social media platforms and proposes a method to automatically filter them. While the topic is relevant and interesting, there are several aspects of the article that require critical analysis.
One potential bias in the article is its focus on spam comments without considering other types of problematic comments, such as hate speech or misinformation. By solely focusing on spam comments, the article may overlook other important issues that can also negatively impact online communities.
The article claims that spam comments aim to promote content or services with little rating or unfamiliar products. However, it does not provide any evidence or examples to support this claim. Without supporting evidence, this claim remains unsupported and lacks credibility.
Additionally, the article mentions YouTube as an example of a platform that allows users to eliminate spam comments through downvoting. While this mechanism exists on YouTube, it fails to acknowledge that downvoting alone may not be sufficient to address the issue of spam comments effectively. There are instances where legitimate comments receive downvotes due to disagreement or bias, which can lead to censorship and suppression of diverse opinions.
Furthermore, the article states that their approach involves eliminating comments with discontinuous text, inadequate text, and vulgar language. While these criteria may help identify some spam comments, they do not necessarily capture all forms of spam. Spam can also include irrelevant or repetitive content that is unrelated to the specific context of a post. The article does not address these aspects adequately.
The article mentions using natural language processing techniques and machine learning algorithms for detecting spam comments but does not provide details about these methods or their effectiveness. Without further information or evidence of their performance, it is difficult to assess the reliability and accuracy of their proposed system.
Moreover, the article lacks exploration of counterarguments or alternative approaches for addressing spam comments. It would have been beneficial to discuss potential challenges in implementing their proposed system or consider different perspectives on the issue.
There is no indication of potential risks or limitations associated with their approach. It is important to consider the ethical implications of automated content filtering, such as the risk of false positives or unintended censorship. These considerations are crucial in ensuring a balanced and fair approach to addressing spam comments.
Overall, the article presents an interesting topic but lacks sufficient evidence, explores limited perspectives, and fails to address potential risks and limitations. A more comprehensive analysis would have provided a more balanced and informative discussion on the identification of spam comments using natural language processing techniques.