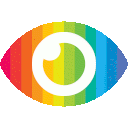
1. Glioblastoma (GBM) is a deadly brain tumor with short overall survival (OS) time, and accurate pre-operative prognosis is desired for personalized treatment.
2. Current machine learning-based studies predict OS time based on pre-operative MR images, but do not consider tumor genotype information, which is only available after craniotomy.
3. This article proposes a deep learning method that uses multimodal MR images to predict OS time and derive genotype-related features, improving the accuracy of personalized prognosis.
The article titled "Pre-operative Overall Survival Time Prediction for Glioblastoma Patients Using Deep Learning on Both Imaging Phenotype and Genotype" discusses a new deep learning-based method for predicting overall survival (OS) time in glioblastoma (GBM) patients. The authors propose a multi-task convolutional neural network (CNN) that can derive genotype-related features from pre-operative multimodal MR images of brain tumor patients to guide OS time prediction.
One potential bias in this article is the focus on deep learning as the primary method for predicting OS time. While deep learning has shown promise in various medical imaging applications, it is important to consider other predictive models and methods as well. The article does not discuss alternative approaches or compare the performance of deep learning with other techniques, which limits the scope of the analysis.
Another potential bias is the limited sample size used to validate the proposed method. The dataset consists of only 120 GBM patients, which may not be representative of the entire population. A larger and more diverse dataset would provide more robust results and increase generalizability.
Additionally, the article does not address potential limitations or challenges associated with using genotype information for OS prediction. It is mentioned that genotype information is unavailable until craniotomy, but there may be other factors that affect the accuracy of genotype prediction or its relationship with OS time. Further exploration of these limitations would provide a more comprehensive analysis.
The article also lacks discussion on potential risks or ethical considerations associated with using deep learning algorithms for personalized prognosis. There are concerns about privacy, data security, and potential biases in algorithmic predictions that should be addressed when implementing such methods in clinical practice.
Furthermore, while the article mentions that tumor genotype has a strong relationship with OS time, it does not provide evidence or references to support this claim. Including relevant studies or research findings would strengthen the argument and provide a more solid foundation for the proposed method.
Overall, while the article presents an interesting approach for predicting OS time in GBM patients, it has several limitations and biases that should be addressed. A more comprehensive analysis, including a larger sample size, consideration of alternative methods, discussion of limitations and risks, and supporting evidence for claims, would enhance the credibility and applicability of the proposed method.