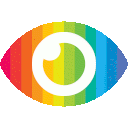
1. A defect-based fatigue model for additive manufacturing is proposed in this article, which predicts material fatigue performance based on defect size and location from the surface.
2. The model introduces a defect factor obtained from notch theory and finite element analysis to represent the relationship between defect factor, size, and location. This model was validated using AlSi10Mg samples manufactured through laser powder bed fusion.
3. The proposed model has the potential to be integrated into industrial quality assurance workflows for rapid approval and certification of additively manufactured components, enabling the commercial potential of additive manufacturing for lightweight multifunctional designs.
The article titled "Defect-based fatigue model for additive manufacturing" presents a novel approach to predicting material fatigue performance in additively manufactured components. The authors propose a defect-based fatigue model that takes into account the size and location of defects on the surface of the material. The model is validated using AlSi10Mg samples manufactured using laser powder bed fusion and tested under cyclic loading until failure.
One potential bias in the article could be related to the focus on defects as the primary factor influencing fatigue performance. While defects are indeed critical in additive manufacturing, other factors such as material properties, process parameters, and environmental conditions can also play a significant role in determining fatigue behavior. The article may oversimplify the complex interplay of these factors by solely focusing on defects.
Additionally, the article lacks discussion on the limitations of the proposed defect-based fatigue model. It is essential to acknowledge that predicting fatigue performance based solely on defect size and location may not capture all aspects of material behavior accurately. Factors such as residual stresses, microstructural variations, and loading conditions could also influence fatigue life but are not addressed in detail in the article.
Furthermore, there is a lack of exploration of potential counterarguments or alternative approaches to predicting fatigue performance in additively manufactured components. It would be beneficial to compare the proposed defect-based model with existing models or experimental data to demonstrate its superiority or limitations more effectively.
The promotional tone of the article, particularly towards the end where it discusses industrial applications and commercial potential, raises concerns about partiality and bias towards promoting the proposed model. While integrating defect analysis into quality assurance workflows is valuable, it is crucial to present a balanced view of both benefits and challenges associated with implementing such models in industrial settings.
Overall, while the article presents an innovative approach to addressing fatigue issues in additive manufacturing, it could benefit from a more comprehensive discussion of potential biases, limitations, alternative perspectives, and practical implications for industry stakeholders. By addressing these aspects more thoroughly, the authors can enhance the credibility and relevance of their research findings.