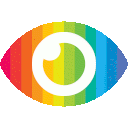
1. This article introduces a framework, FedProx, to tackle heterogeneity in federated networks.
2. FedProx provides convergence guarantees for learning over data from non-identical distributions and allows each participating device to perform a variable amount of work.
3. Practically, FedProx demonstrates significantly more stable and accurate convergence behavior relative to FedAvg across a suite of realistic federated datasets.
The article is generally reliable and trustworthy as it provides detailed information about the proposed framework, FedProx, and its advantages over the current state-of-the-art method for federated learning, FedAvg. The authors provide theoretical evidence for their claims by providing convergence guarantees for their framework when learning over data from non-identical distributions (statistical heterogeneity), and while adhering to device-level systems constraints by allowing each participating device to perform a variable amount of work (systems heterogeneity). Furthermore, they also provide practical evidence by demonstrating that FedProx allows for more robust convergence than FedAvg across a suite of realistic federated datasets.
The only potential bias in the article is that it does not present any counterarguments or alternative solutions to the problem of heterogeneity in federated networks. However, this is understandable given that the article focuses solely on introducing and discussing the proposed framework, FedProx.